The inferential cognitive geometry of language and action planning: Common computations?
The efficiency and flexibility with which humans generate meaning during language comprehension (or production) is remarkable. How does our brain do it? To move beyond the many extant attempts to address this big quest, BQ5 will treat linguistic inference as an instance of an advanced generative planning solution to the multi-step, sequential choice problems that we also face in other cognitive domains (e.g. chess, foraging and spatial navigation). Thus, BQ5 anticipates making unique progress in unravelling the mechanisms of fast, flexible and generative linguistic inference by leveraging recent major advances in our understanding of the representations and computations necessary for sequential model-based action planning. This approach will also lead us to revise current dual-system dogma’s in non-linguistic domains, that have commonly over-focused on the contrast between a cognitive (flexible, but slow) and a habitual (fast, but inflexible) system: The current quest will encourage the integration of so-called ‘cognitive habits’ and their associated cognitive map-related neural mechanisms into theoretical models of both linguistic and non-linguistic inference.
We will leverage current rapid conceptual and methodological progress in our understanding of other cognitive systems, such as the ‘cognitive mapping’ mechanisms for action planning (Behrens et al., 2018; Bellmund et al., 2018), as well as predictive inference in perception (Martin, 2016; Martin, 2020), to advance our understanding of how we generate meaning in the state space of language. In non-linguistic problems, the goal state is a function of the reward that is to be maximized. In the linguistic problem that we consider here, the goal state is the compositional meaning that needs to be generated during comprehension and production. Leveraging the recently developed approaches to understand perceptual inference and action planning, we will contribute unique advances in our understanding of the neural code and computations that underlie the unbounded combinatoriality of language, i.e., the ease with which we can generate meaning.
People involved
Steering group
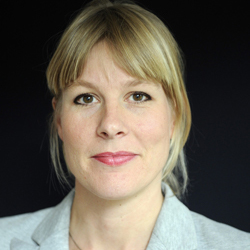
Prof. dr. Roshan Cools
PI / Coordinator BQ5
Profile page

Dr. Xiaochen Zheng
Coordinating Postdoc BQ5
Profile page
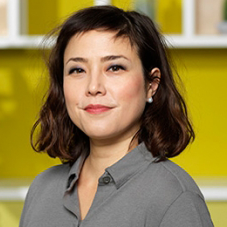
Dr. Andrea Martin
PI / Coordinator BQ5
Profile page
Team members
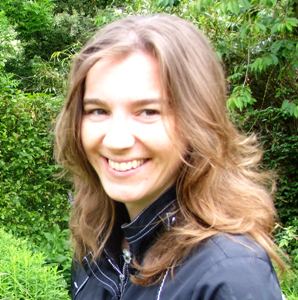
Dr. Hanneke den Ouden
PI
Profile page

Dr. Silvy Collin
PI
Profile page
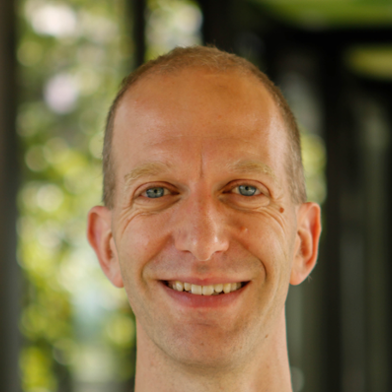
Dr. Stefan Frank
Coordinator BQ1
Tenure track researcher
Profile page

Prof. dr. Peter Hagoort
Programme Director
PI / Coordinator BQ2
Profile page
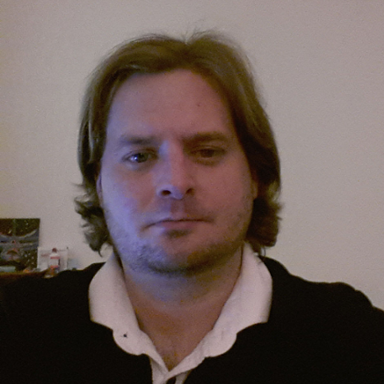
Dr. Ashley Lewis
Coordinating Postdoc BQ2
Profile page
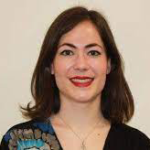
Dr. Ioanna Zioga
Postdoc
Profile page
Dr. Marius Braunsdorf
Postdoc

Dr. Roel Willems
PI
Profile page

Prof. dr. Ivan Toni
PI / Coordinator BQ3
Profile page

Prof. dr. Iris van Rooij
PI
Profile page
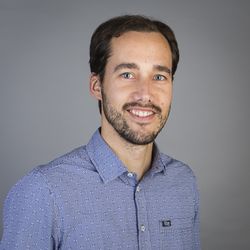
Dr. Bob van Tiel
Postdoc
Profile page
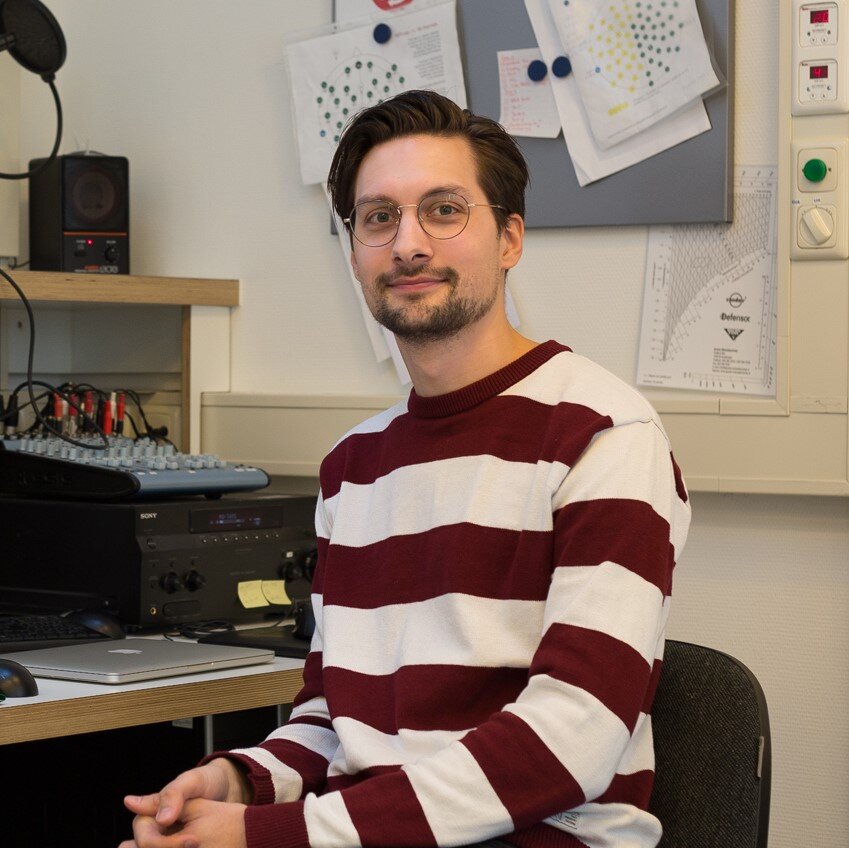
Dr. Rene Terporten
Postdoc
Profile page

Dr. Marieke Woensdregt
Postdoc
Profile page
PhD Candidates
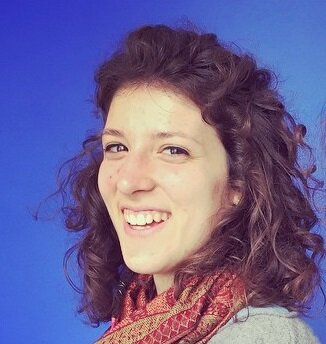
Elena Mainetto
PhD Candidate
Profile page
Collaborators
Dr. Mark Blokpoel
Dr. Monique Flecken
Dr. Naomi de Haas
Dr. Saskia Haegens
Dr. Yingying Tan
Alumni
Dr. Branka Milivojevic – Postdoc
Dr. Mona Garvert
Research Highlights (2021)
Highlight 1
Generalization and representation of novel compositional word meanings
Team members: Xiaochen Zheng, Mona Garvert, Hanneke den Ouden, Roshan Cools
The ability to generalize previously learned information to novel situations is key for adaptive behavior. The team investigated the neural mechanisms underlying the ability to infer novel compositional word meanings using a novel behavioral paradigm in fMRI. Results suggest that generative inference in language recruits a domain-general network shared with action planning, compositional vision and constructive relational memory, while the newly inferred meanings are represented in more language-specific regions.
Participants were taught the meaning of artificial compositional words from an artificial language comprising extant stems (“good”) and novel affixes (“kla”). The meaning of the compositional words depended on the position of the novel affix (“goodkla = bad”, “klahorse = pony”). They were then asked to infer the meaning of novel compositional words (“whitekla =?”, “klacat =?”) which were either congruent or incongruent with the rule (“klawhite” is incongruent because a small version of “white” does not exist). To do this, they had to generalize the sequential order rule they inferred during training (e.g., if kla occurs after the stem, then it reverses the meaning of the stem). During fMRI, participants performed a semantic priming task in which the novel words served as congruent or incongruent primes (“whitekla”) and their synonyms (“black”) served as targets. Faster responses on congruent than incongruent targets show that people are able to generate novel compositional meanings on the fly, successfully inferring meanings of congruent versus incongruent words. Neural repetition suppression effects at target were greater when primed with congruent than incongruent words in the left inferior frontal gyrus, suggesting the novel meanings to be derived at this linguistic “building” hub. Analysis of congruent versus incongruent prime-related activity revealed a broad frontal-parietal network, including hippocampus, the brain area commonly associated with the generalization process of abstract, generalizable, structural relationships.

The current project investigates structural inference in language and leverages knowledge from vision, memory and planning. It not only extends our understanding of the flexible and efficient nature of language processing – the key question addressed by BQ5 – , but also probes its possibly shared mechanisms with other cognitive domains. The current project is challenging because of the different languages spoken by and common conceptual misalignment between the psycholinguists versus neuroscientists on learning and decision making. Nevertheless, through active, resilient and well-coordinated team science, an integrative novel design, unique ideas and preliminary advance in understanding were achieved not otherwise possible.
Highlight 2
Parallel cognitive maps for short-term statistical and long-term semantic relationships in the hippocampal formation
Team members: Xiaochen Zheng, Roshan Cools, Mona Garvert, Martin Hebart, Raymond Dolan, Christian Doeller
The hippocampal-entorhinal system uses cognitive maps to represent spatial knowledge and other types of relational information. We investigated how the hippocampal formation handles the embedding of stimuli in multiple relational structures that differ vastly in terms of their mode and timescale of acquisition (e.g., semantic similarities learned over the course of one’s lifetime versus transitions experienced over a brief timeframe in an experimental setting).
We reanalysed functional magnetic resonance imaging (fMRI) data from Garvert et al. (2017) that had previously revealed an entorhinal map which coded for newly learnt statistical regularities. We used a triplet odd-one-out task to construct a semantic distance matrix for presented items and applied fMRI adaptation analysis to show that the degree of similarity of representations in bilateral hippocampus decreases as a function of semantic distance between presented objects. Importantly, while both maps localize to the hippocampal formation, this semantic map is anatomically distinct from the originally described entorhinal map. This finding supports the idea that the hippocampal-entorhinal system forms parallel cognitive maps reflecting the embedding of objects in diverse relational structures.

This work contributes significantly to current theorizing about how our brain organizes knowledge of the world, and imposes new constraints on the cognitive computations the brain can perform to make novel inferences and support flexible behaviour. This project is closely related to the original BQ5 proposal on map-like encoding for integrated cognitive representation. Knowledge gained from this project also supports multiple BQ5 projects such as meaning representation and structural inference (SP1 & SP5). This work concerns interdisciplinary team work (e.g., language, memory, and decision making) from three international institutes (MPI Leipzig, UCL, Donders). We also took an open science approach, re-using an existing fMRI dataset to address a novel question.
Highlight 3
Computational modelling to explain flexible linguistic inference
Team members: Marieke Woensdregt, Andrea E. Martin, Iris van Rooij and Mark Blokpoel
Language comprehension involves fast and flexible inference (e.g., we can infer the meaning of a novel phrase like “mask-shaming” on first encounter). This requires two foundational abilities: (i) understanding of compositionality, and (ii) integration of context. In this project, we use computational modelling to develop an explanation of the cognitive process that combines these two abilities in order to infer linguistic meaning
We take meaning inference of novel compound words as a test case (e.g., anderhalvemetersamenleving in Dutch, which means “one-and-a-half metre society”). We are in the process of developing a computational-level model of this inference process by building on work by Blokpoel et al. (2019), which models the cognitive ability of coming up with novel hypotheses to explain a given observation, by finding analogical matches between (transformed) representations of the observation and (transformed) representations of knowledge. Figure 9 illustrates how this computational model could be adapted for the case of inferring the meaning of novel compound words, where various constraints (lexical meaning, grammar, and background knowledge) could influence either which higher-level representations are formed, or which get selected as a plausible interpretation (through a process of inference-to-the-best-explanation).
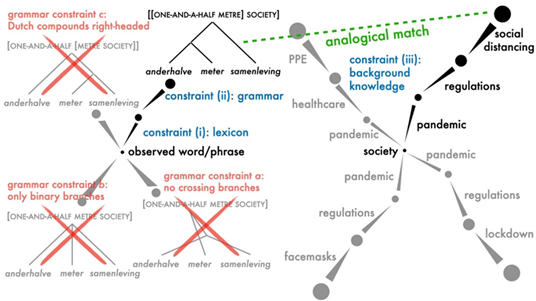
Language is a dynamic phenomenon, and humans are remarkably flexible in using it. This project contributes theoretical work that aims to explain the cognitive processes that make this possible, by bringing together knowledge from linguistics, computational complexity theory and systems neuroscience, through computational modelling. Interaction with the BQ5 team has helped sharpen our formulation of the explanandum for this subproject. Furthermore, this subproject benefits greatly from discussion and collaboration within the research groups of PIs Andrea Martin (Language and Computation in Neural Systems) and Iris van Rooij (Computational Cognitive Science).