The nature of the mental lexicon: How to bridge neurobiology and psycholinguistic theory by computational modelling?
This Big Question addresses how to use computational modelling to link levels of description, from neurons to cognition and behaviour, in understanding the language system. Focus is on the mental lexicon and the aim is to characterize its structure in a way that is precise and meaningful in neurobiological and (psycho)linguistic terms. The overarching goal is to devise causal/explanatory models of the mental lexicon that can explain neural and behavioural data. This will significantly deepen our understanding of the neural, cognitive, and functional properties of the mental lexicon, lexical access, and lexical acquisition.
The BQ1 team takes advantage of recent progress in the understanding of modelling realistic neural networks, improvements in neuroimaging techniques and data analysis, and developments in accounting for the semantic, syntactic and phonological properties of words and other items stored in the mental lexicon. Using one common notation ‒high-dimensional numerical vectors‒ neurobiological and computational (psycho)linguistic models of the mental lexicon are integrated and methods are developed for comparing model predictions to large-scale neuroimaging data.
BQ1 thus comprises three main research strands, respectively focusing on models of lexical representation, models of neural processing, and methods for bridging between model predictions and neural data. It is taken into account that lexical items rarely occur in isolation but form parts of (and are interpreted in the context of) sentences and discourse. Moreover, the BQ1-team refrains from prior assumptions about what the lexical items are, that is, lexical items do not need to be equivalent to words but may be smaller or large units.
Thus, the Big Question is tackled from three directions:
(i) by investigating which vector representations of items in the mental lexicon are appropriate to encode their linguistically salient (semantic, combinatorial, and phonological) properties;
(ii) by developing neural processing models of access to, and development of, the mental lexicon; and
(iii) by designing novel evaluation methods and accessing appropriate data for linking the models to neuroimaging and behavioural data.
The BQ1 endeavour is inherently interdisciplinary in that it applies computational research methods to explain neural, behavioural, and linguistic empirical phenomena. One of its main innovative aspects consists of bringing together neurobiology, psycholinguistics, and linguistic theory (roughly corresponding to different levels of description of the language system) using a single mathematical formalism; a feat that requires extensive interdisciplinary team collaboration. Thus, BQ1 integrates questions of a Linguistic, Psychological, Neuroscientific, and Data-analytic nature.
People involved
Steering group
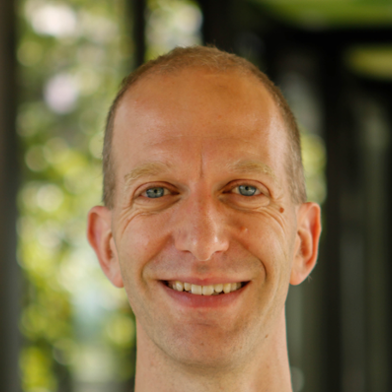
Dr. Stefan Frank
Coordinator BQ1
Tenure track researcher
Profile page
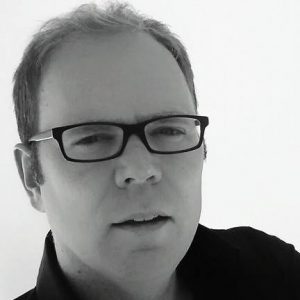
Dr. Jelle Zuidema
Tenure track researcher
Profile page
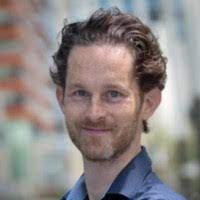
Prof. dr. Marcel van Gerven
PI
Profile page

Dr. Hartmut Fitz
Profile page
Team Members
Prof. dr. Rens Bod
PI
Profile page

Prof. dr. Mirjam Ernestus
PI
Profile page
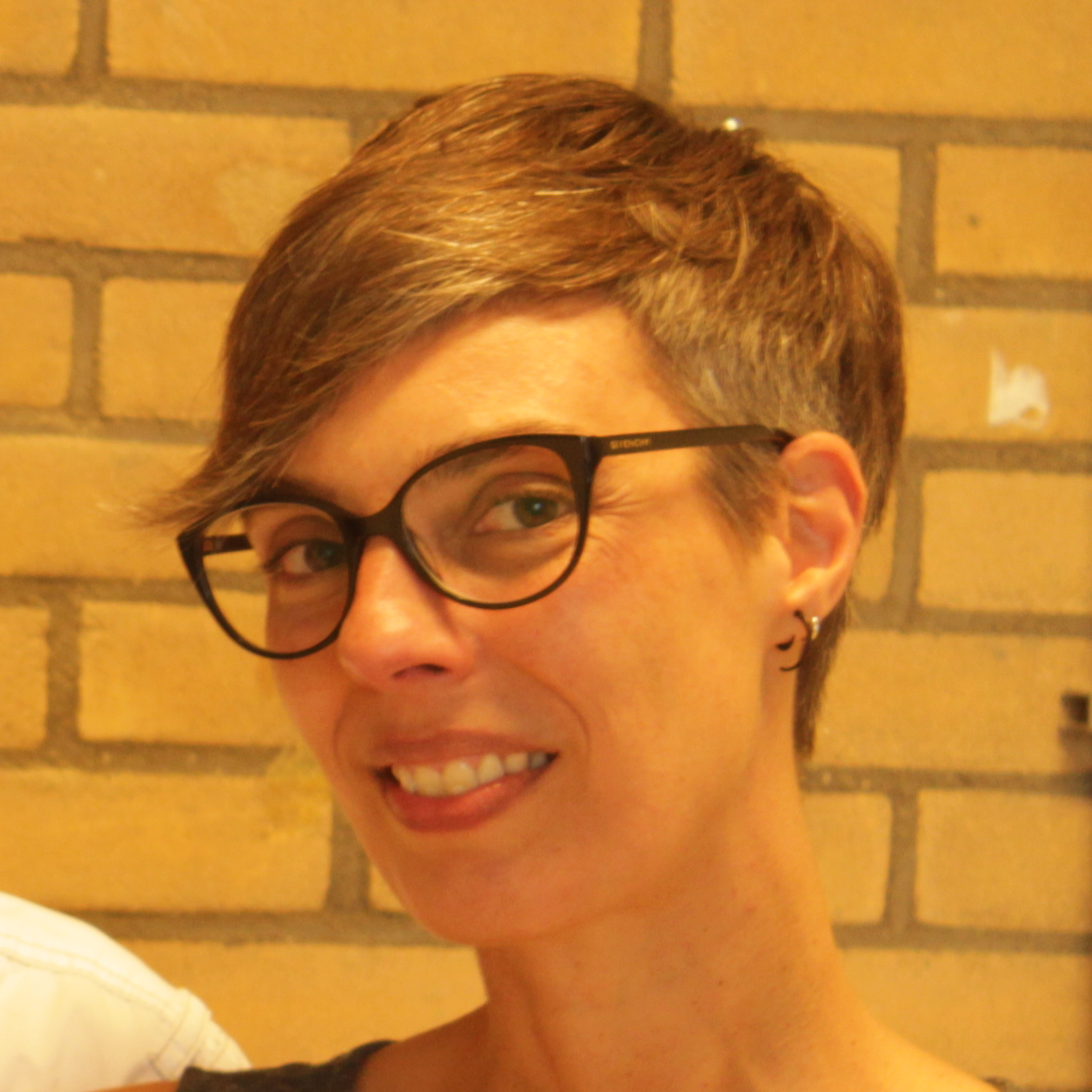
Dr. Raquel Fernández
PI
Profile page

Prof. dr. Peter Hagoort
Programme Director
PI / Coordinator BQ2
Profile page
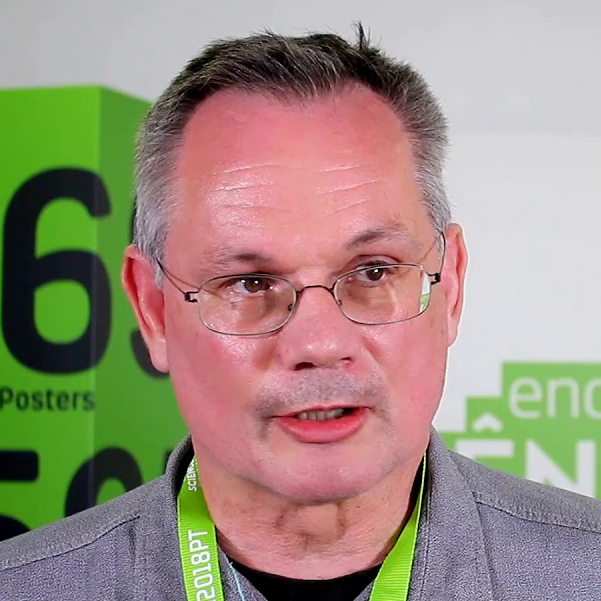
Dr. Karl-Magnus Petersson
PI
Profile page
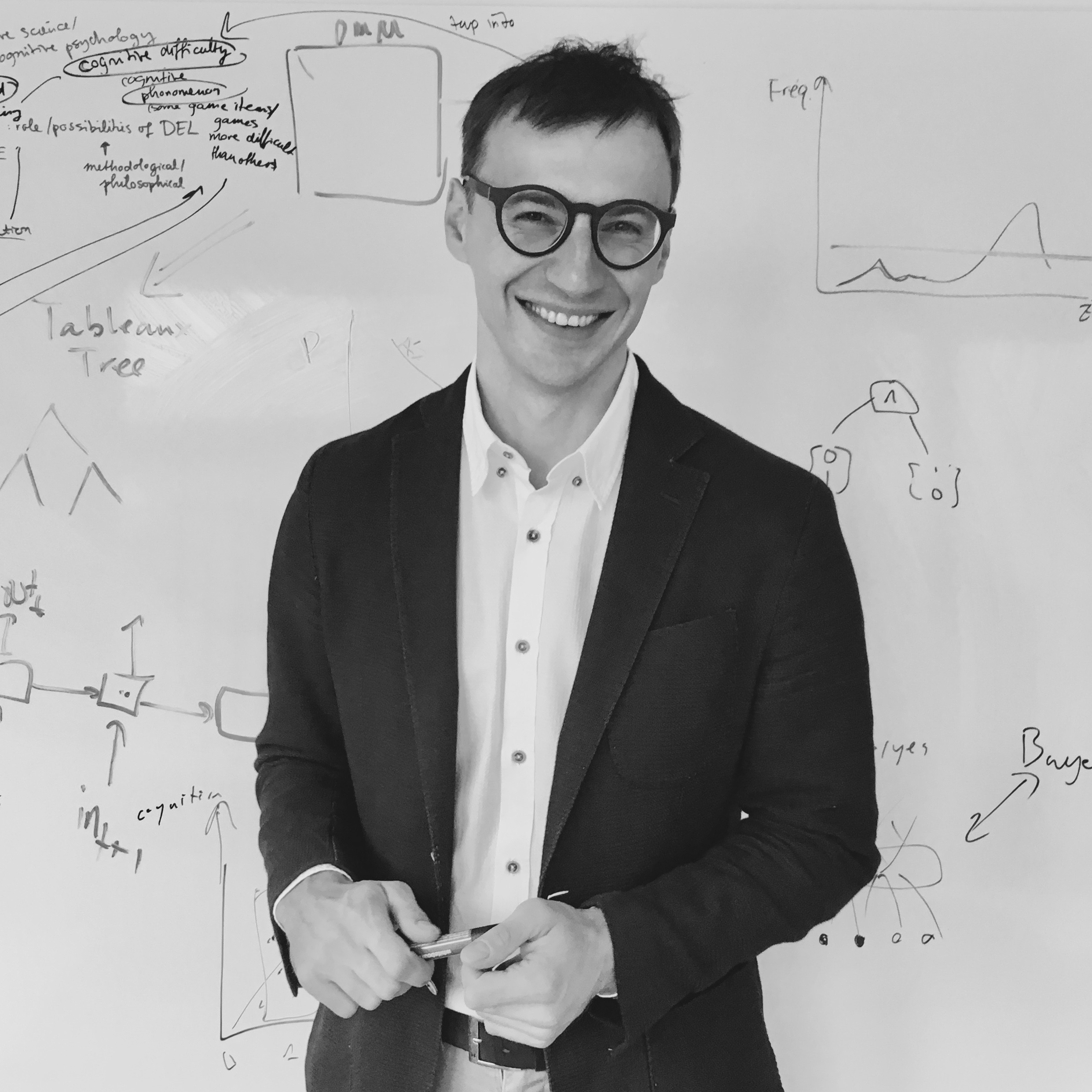
Dr. Jakub Szymanik
PI
Profile page

Prof. dr. Robert van Rooij
PI
Profile page

Dr. Tamar Johnson
Postdoc
Profile page
PhD Candidates
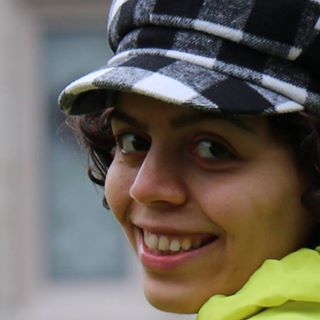
Samira Abnar
PhD Candidate
Profile page
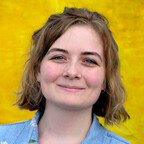
Marianne de Heer Kloots
PhD Candidate
Profile page

Alessio Quaresima
PhD Candidate
Profile page
Collaborators
Dr. Luca Ambrogioni
Dr. Julia Berezutskaya
Dr. Louis ten Bosch
Dr. Renato Duarte
Dr. Umut Güçlü
Prof. dr. Abigail Morrison
Dr. David Neville
Dr. Roel Willems
Alumni
Lisa Beinborn – Postdoc
Hartmut Fitz – Postdoc
Dieuwke Hupkes – PhD
Alessandro Lopopolo – PhD
Danny Merkx – PhD
Joe Rodd – PhD
Chara Tsoukala – PhD
Marvin Uhlmann – PhD
Research Highlights (2022)
Highlight 1
Visually grounding word embeddings to better capture human semantic knowledge
Danny Merkx, Stefan Frank and Mirjam Ernestus
Distributional semantic models capture word-level meaning that is useful in many natural language processing tasks and have even been shown to capture cognitive aspects of word meaning. The majority of these models are purely text based, even though the human sensory experience is much richer. In this paper we create visually grounded word embeddings by combining English text and images and compare them to popular text-based methods, to see if visual information allows our model to better capture cognitive aspects of word meaning. Our analysis shows that visually grounded embedding similarities are more predictive of the human reaction times in a large semantic priming experiment than the purely text-based embeddings.

The visually grounded embeddings also correlate well with human word similarity ratings. Importantly, in both experiments we show that the grounded embeddings account for a unique portion of explained variance, even when we include text-based embeddings trained on huge corpora. This shows that visual grounding allows our model to capture information that cannot be extracted using text as the only source of information.
The coloured bars indicate the proportion of explained variance (R2) on eight data(sub)sets by four word-embedding models: our Visual Grounded Embeddings (VGE) and three well known text-based models. The grey-scale bars on top of the R2 of the text-based models indicate the semi-partial R2 and their significance (* p < .05, ** p < .01, *** p < .001) of the VGEs after controlling for the variance explained by that text-based model. Left panel: models trained on the MSCOCO dataset of image-caption pairs. Right panel: models trained on very large text-only datasets.
Highlight 2
The Tripod neuron: a minimal structural reduction of the dendritic tree
Alessio Quaresima, Hartmut Fitz, Renato Duarte, Dick van den Broek, Peter Hagoort, Karl Magnus Petersson
Neuron models with explicit dendritic dynamics have shed light on mechanisms for coincidence detection, pathway selection and temporal filtering. However, it is still unclear which morphological and physiological features are required to capture these phenomena. In this work, we introduce the Tripod neuron model and propose a minimal structural reduction of the dendritic tree that is able to reproduce these computations. The Tripod is a three-compartment model consisting of two segregated passive dendrites and a somatic compartment modelled as an adaptive, exponential integrate-and-fire neuron. It incorporates dendritic geometry, membrane physiology and receptor dynamics as measured in human pyramidal cells.
We characterize the response of the Tripod to glutamatergic and GABAergic inputs and identify parameters that support supra-linear integration, coincidence-detection and pathway-specific gating through shunting inhibition. Following NMDA spikes, the Tripod neuron generates plateau potentials whose duration depends on the dendritic length and the strength of synaptic input. When fitted with distal compartments, the Tripod encodes previous activity into a dendritic depolarized state. This dendritic memory allows the neuron to perform temporal binding, and we show that it solves transition and sequence detection tasks on which a single-compartment model fails. Thus, the Tripod can account for dendritic computations previously explained only with more detailed neuron models or neural networks. Due to its simplicity, the Tripod neuron can be used efficiently in simulations of larger cortical circuits.
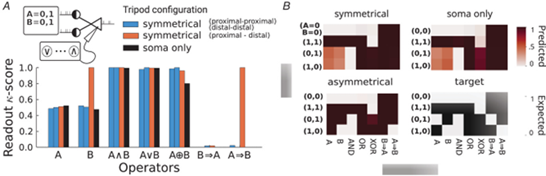
Figure 2. Asymmetric dendrites enhance separability of logical operations.
A, Cohen’s kappa-score accuracy of linear readout classifiers on logical operators for symmetric, asymmetric and soma-only models. The dendritic configurations are proximal–proximal and distal–distal (blue), proximal–distal (orange) and soma-only (black). B, Shade of red indicates the average predicted truth-value for each input condition (y-axis), operator (x-axis) and dendritic configuration (top and left panels). Black and white table (bottom-right) indicates the expected truth-values. For example the AND operator for symmetric dendrites shows dark red (true) for condition A = 1, B = 1, and white for all the remaining conditions, corresponding to the target truth-values.